Summary. The idea of business process reengineering is making a comeback, this time driven by artificial intelligence (AI). In the 1990s, the implementation of enterprise resource planning systems and the internet allowed companies to make changes to broad business.
In the 1990s, business process reengineering was all the rage: Companies used budding technologies such as enterprise resource planning (ERP) systems and the internet to enact radical changes to broad, end-to-end business processes. Buoyed by reengineering’s academic and consulting proponents, companies anticipated transformative changes to broad processes like order-to-cash and conception to commercialization of new products.
But while technology did bring major updates, implementations often failed to live up to the sky-high expectations. For example, large-scale ERP systems like SAP or Oracle provided a useful IT backbone to exchange data, yet also created very rigid processes that were hard to change past the IT implementation. Since then, process management typically involved only incremental change to local processes — Lean and Six Sigma for repetitive processes, and Agile Lean Startup methods for development — all without any assistance from technology.
Now, a version of this idea is making a comeback in some companies, and we expect to see it in more. It will require not only an appreciation and understanding of AI but also a renewed appreciation of business processes as a structure for improving work. As AI emerges as a universally applicable, general-purpose technology, it appears increasingly possible to enable the kind of radical redesign of business processes originally envisaged by proponents of reengineering.
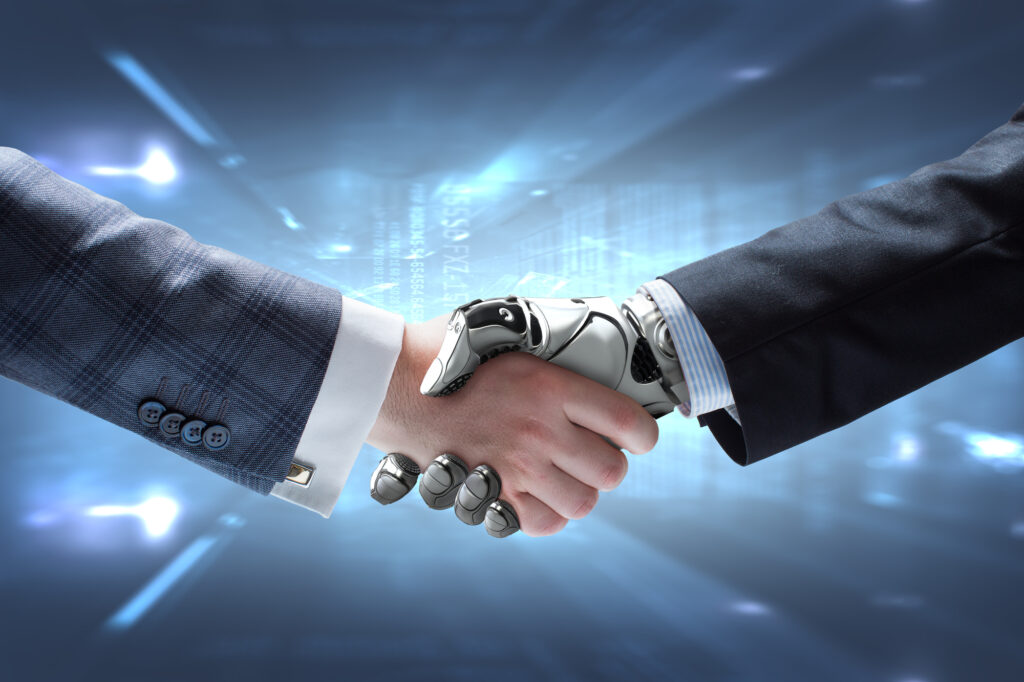
Updating Reengineering
The technologies enabling reengineering in the 90s were primarily transactional and communications-based. They enabled efficient data capture and transfer within and across organizations. AI, on the other hand, enables better, faster, and more automated decisions. In essence, most AI deployments in large organizations involve learning from large datasets to make a prediction or classification, which in turn helps businesses with making better operational decisions. Better operational decisions, in turn, enhance efficiency by producing better outcomes. A key difference is that present AI systems are a true general-purpose technology and have brought about dramatic changes not just in production planning and control, but also in visual image recognition and inspection, autonomous operations, and generating new content.
While the methods fueling this growth of AI have been around for decades, the cost of implementing them has dropped precipitously. Previously the domain of data scientists only, modern AI-based solutions is now mature enough to be offered “off the shelf,” greatly lowering the technical barriers to entry. Falling computing costs — driven by the wide availability of the cloud, the growth of low-cost bandwidth, and the reduced cost of sensors — have drastically lowered the price of model-driven prediction. AI-based decisions can also be included within the wider context of automation. Technologies such as robotic process automation (RPA) help to structure the flow of work and automate information-intensive back-office processes. RPA is rule-based, which limits its ability to employ data-based decisions. But combined with machine learning as “intelligent process automation,” it can deal with much a greater variety of tasks.
This AI-driven reengineering is already taking place. Banks are using it to transform wealth management advice for clients. Insurance companies are using AI to make client onboarding and underwriting much easier, and automating claims estimates for auto and home damage with deep learning analysis of photos taken by the insured. Industrial firms are reshaping maintenance and engineering processes. Even in health care, where there is considerable research on AI but much less clinical adoption, diagnosis and treatment are being reshaped by AI-based telemedicine in some countries.
All of this has important ramifications for the way we use AI, how work is being performed, and how companies are being organized. To take advantage of these potential benefits, companies need to bring back an end-to-end process perspective on their businesses and think carefully about how AI can transform them. In essence, companies need to explore where they are generating sufficient data to extract patterns that could be used to support operational decisions.
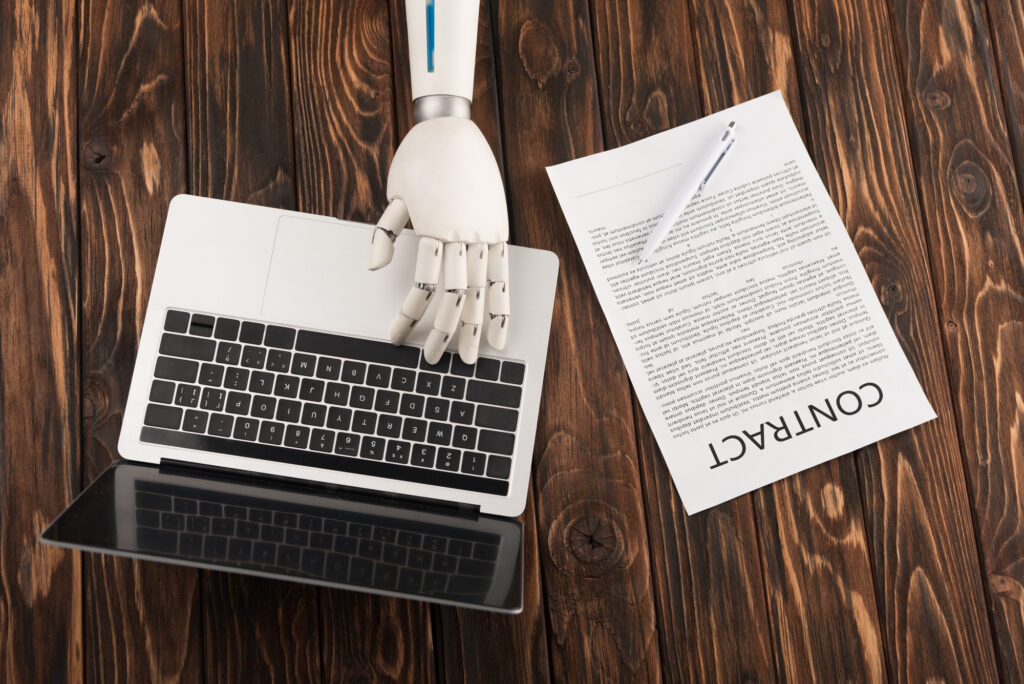
AI Drives Process Reengineering
As AI brings new capabilities to a business process, companies need to rethink what tasks are needed, in what frequency, and who does them. When AI is accompanied by partial automation, companies also need to decide what humans will do and what machines will do in their processes. Most AI applications to date seek to improve a given task. But this is missing the larger picture; smart companies are viewing the introduction of AI as the rationale for a new look at end-to-end processes.
At its most basic level, process analysis often involves a mix of constraints and opportunities. For example, at DBS Bank in Singapore, the manager of transaction surveillance (anti-money laundering and fraud detection) said in an interview they were frustrated at the high false positive levels identified by the rule-based system required by banking regulators. That is an unavoidable constraint on the process, but he saw an opportunity to use AI to predict and score the fraud risk of each positive result using machine learning. Transactions with low fraud probabilities could simply be put in a “cooler” for several months to see if they recurred with the same customer. Machine-learning-based AI systems to detect outliers are well-established in the fraud detection arena. But when the machine learning system was combined with a new workflow platform and a relationship network analytics system (to identify fraud network members), the productivity of surveillance analysts increased by a third.
Another good example is at Shell, where one of us (Jeavons) leads AI initiatives. Shell has long been a process-focused company and is presently engaged in a significant AI initiative in areas like supply chain, operations, and maintenance. As part of this, Shell is reengineering its work processes.
For example, consider the work of monitoring and inspection at energy and chemical plants, pipelines, offshore facilities, and wind and solar farms. This work used to be exclusively done in person by inspectors and maintenance technicians, but AI can ease that constraint. Now many low-value-adding inspection tasks can instead be done remotely by robots and drones. Some Shell facilities are so large that it would previously have taken years to inspect everything manually — now drones and robots are being introduced to automate these processes and help shorten the cycle time.
As a result of these changes, inspectors and maintenance technicians can now rethink their day-to-day work. They can focus on higher-value activities such as prioritizing projects or, if they’re on-site, performing more advanced verification. At the same time, new tasks are emerging, such as annotation for images to improve inspection algorithms or managing the training processes for the thousands of machine learning models now working in production. What were previously physical work processes are now managed by multidisciplinary teams doing largely digital tasks.
This shift involved some resistance. At first, it was hard to convince the inspectors, but gradually they are being persuaded as they were shown that the processing of the images provides similar accuracy in much less time. Furthermore, Shell is engaging these engineers in rethinking their work processes with the remote surveillance centers, empowering them to drive the change.
Shell is finding that this process of AI-enabled reengineering is becoming a permanent way of operating. Each project may take only a year or two, but the more they use digital, data, and AI to redesign processes, the more they see opportunities for going further. This is particularly important as the company is transforming to become a net-zero emissions energy company.
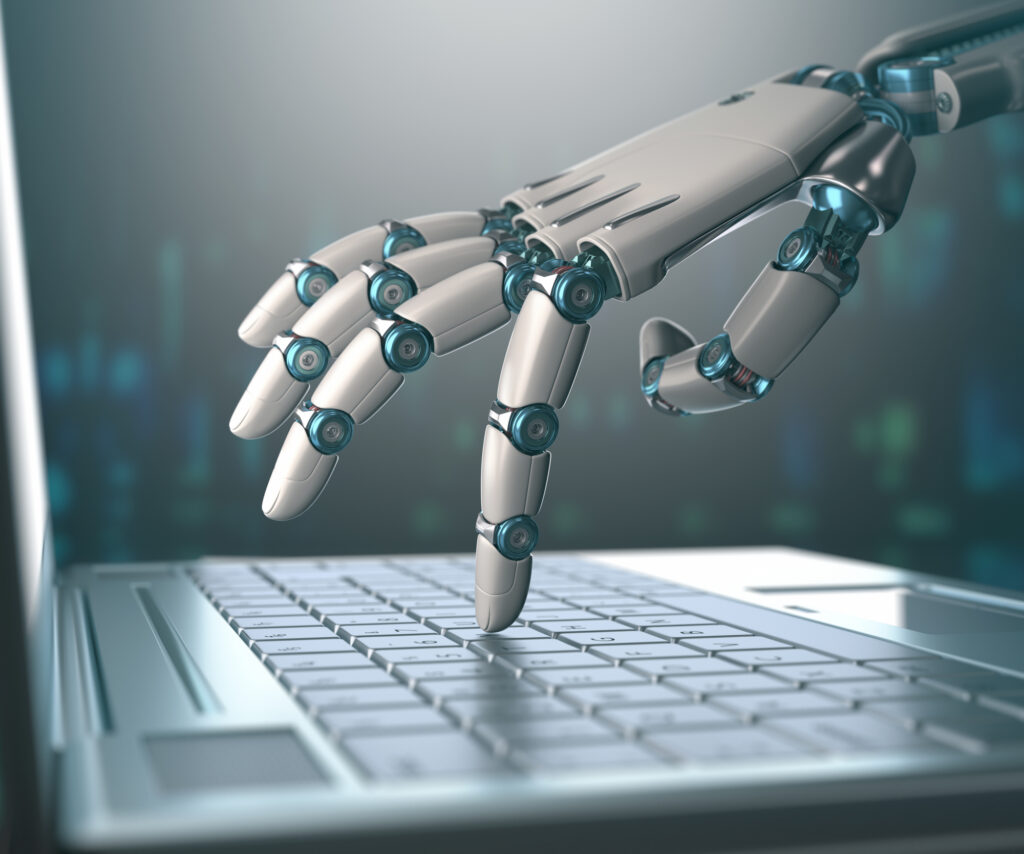
Who Should Lead AI-Enabled Process Change?
Process improvement has traditionally solely been the domain of operations managers. For that reason, it has been somewhat rare for organizations to have an explicit reengineering initiative in conjunction with their AI projects. To truly leverage the power of AI, process design and improvement activities should be incorporated within the AI initiative. The most successful such initiatives are increasingly being orchestrated by “product managers” who have the successful deployment of the system, including the needed business changes, as their objective. Shell designates a product owner to manage the business change and a product manager responsible for technical delivery. Some organizations also engage in “design thinking” exercises, which overlap in part with reengineering-style analyses of how workflows and activities need to be redesigned to meet customer or internal needs.
While we have seen multiple instances in which reengineering takes place alongside AI development, not enough organizations yet recognize the need for process change. A more explicit callout of the reengineering role and activities — including high-level design, detailed process flows, measurement of before and after costs and cycle times, and analysis of needed skills and training — would be helpful, whether it is called “reengineering” or not. The activities are too important to the success of AI projects to leave them to chance or an astute manager who remembers the reengineering movement.
Because automation-focused projects have a direct impact on process flows and are more likely than other forms of AI to involve only incremental change, they are more likely to include a formal set of process improvement steps. At Voya Financial, for example, the process improvement group has an automation center of excellence within it, and no automation project takes place without a process improvement effort first. The group’s head told us that automation at the company is as much a process-oriented engagement as a technical one. We’ve found several other companies that combine process improvement and automation, but we’d like to see both more aggressive process change and more powerful AI technologies like machine learning in combination as well.
AI is fast becoming a ubiquitous technology. Once the hype recedes it will become as standard as ERP systems, statistical packages, or even spreadsheets. AI platforms can be used by a much larger pool of companies to reengineer their processes. AI is a means to an end, not an end in itself. Firms that understand how to use it as a new tool in the broader context of process reengineering will arguably get the most from AI in the long run.