Mailchimp launched Content Optimizer earlier this month. This new product uses artificial intelligence (AI) to improve the performance and effectiveness of email marketing campaigns.
Mailchimp’s vast data set allows it to identify common patterns in successful marketing campaigns. Content Optimizer uses these data, machine learning models, and business rules, to predict the quality and effectiveness of email campaigns, and to provide suggestions for improving content, layout, or imagery.
John Wolf, product manager of innovative content at Mailchimp, is leading the effort to develop Content Optimizer. John Wolf, Mailchimp’s product manager for smart content, is leading the effort to create Content Optimizer. Wolf founded Inspector 6, an early startup that was acquired by Mailchimp in 2020. Inspector 6’s technology and experience was a key factor in the development of Content Optimizer.
In an interview, Wolf shared some insights about the development of Content Optimizer. He also discussed how AI will change the future of content.
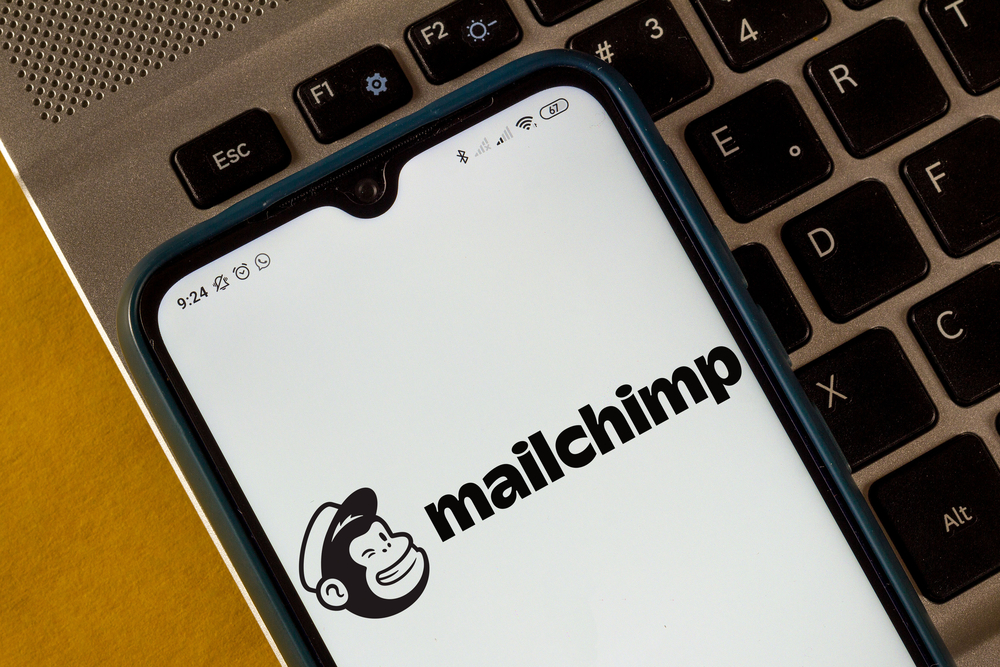
AI content marketing: The future vision
Content Optimizer was born out of pain. Wolf saw the need for AI content marketing when he worked as a chief marketing officer of Intradiem.
Intradiem, like all businesses, needed excellent marketing content. The process was challenging, and evaluating quality and success was subjective.
The creative process was dominated by opinions and little data. Wolf said that the process was laborious, manual, and involved many cycles. “There has to be a better way,” he added.
Machine learning was beginning to have real-world applications in many industries. Wolf began to consider using ML to optimize the content marketing creation process.
The idea was: What if we used machine learning to better understand marketing content? “If the software can understand the story that marketing content tells and how it does it, then it can correlate features to marketing outcomes. It can also start to standardize much of the creative processes and replace opinions with data,” Wolf explained.
Wolf founded Inspector 6 in 2017 to create AI-powered marketing content. Inspector 6 is an AI platform that analyzes content marketing to provide insights and improvements.
Meeting data challenges
As with all machine learning applications, the optimization of marketing content relies on a large amount of high-quality data. Inspector 6’s platform has been successful in certain areas, but only in some.
“Our biggest customer was a large multinational consumer packaged products company with 500 brands and 200 countries. I thought that they would have enough data to fuel my predictive models. Wolf explained that he found out it was not necessarily true. It was harder to provide value for small business owners since their data set for machine learning models was even smaller.
Mailchimp and Inspector 6 had a partnership before Mailchimp bought Inspector 6. Mailchimp sends hundreds of billions of emails every year. Through the partnership, Inspector 6 gained the opportunity to train its models using the vast amount of marketing data Mailchimp holds.
The amount and breadth of data are key to providing a solution such as this. From marketing content to sell products and services to content marketing, newsletters, and everything else in between, some of which are very high performing and others, just as important, low-performing, having that level and breadth data becomes crucial,” Wolf said.
Context is also important for recommendations. Analysts would need to be aware of the campaign’s goal, vertical business, target audience, and other details before they can provide feedback. Machine learning models also require context. Mailchimp has a large number of data from its diverse customer base. This allows it to create machine-learning models which can be used in different contexts.
Wolf explained that the 360 billion emails per year from Mailchimp would be used as a training set. However, you’d need to break it down by context to solve the problem. “So, basically, 360 billion emails become a collection that is context-specific of individual training sets.”
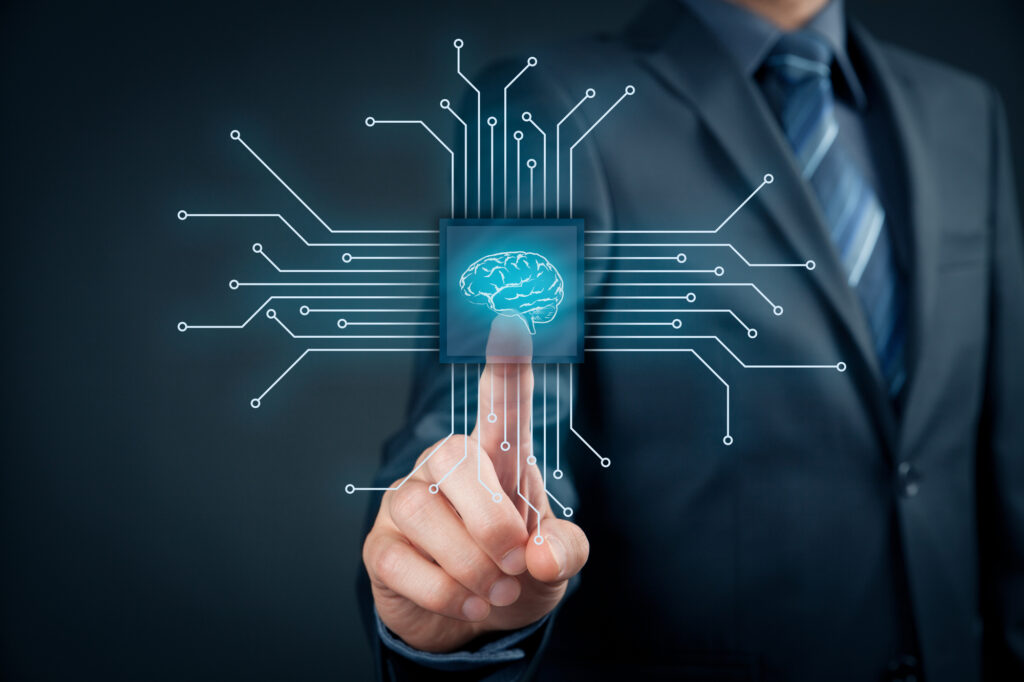
Mailchimp: How to switch?
Mailchimp’s partnership with Inspector 6 led to an acquisition proposal that was a win for both companies.
Mailchimp’s data and infrastructure allowed Inspector 6 to extend its application to an even wider customer base.
Wolf explained that “having gone through the experience and knowing how much information my predictive models would require, and how much Mailchimp already had, I thought that it could be interesting to solve this problem, which I am solving mainly for a few large multinationals, for millions or even tens of thousands of small businesses. This is more in line with my passions,” he said.
Mailchimp, on the other hand, was able to enhance its AI efforts through the acquisition of a proven backend technology stack that predicts marketing campaign outcomes, as well as a team of data scientists and engineers, focused on the intersection of marketing and computer science.
Wolf stated that “Mailchimp had experience in all those areas but to be able to focus on solving the problem at this intersection was what Mailchimp most wanted from a talent perspective.”
Inspector 6’s technology was architected as microservices in Amazon Web Services. The system receives a marketing asset and then the microservices analyze it independently.
Mailchimp uses the Google Cloud Platform. The services needed to be moved from one cloud platform to another. Mailchimp, which was acquired by Google, had already undertaken a major project to move all of its data to Google BigQuery. This cloud-based data store makes it simple to manage large amounts of data and use them for data analysis and machine-learning pipelines. Mailchimp uses GCP’s Dataflow service, which creates dynamic views from real-time data and stored data.
It was much easier to integrate Inspector 6 into Mailchimp’s cloud infrastructure.
Wolf explained that “from a technological standpoint, we moved from one set of microservices into another and it worked quite well.”
The microservices of Inspector 6 are a technology that enables. They are integrated into the backend system of Mailchimp and made available through front-end products. The service began as a reporting service for Mailchimp but evolved into a content insight generator through the front-end product group. The Content Optimizer, which was based on Wolf’s vision for AI-powered marketing campaign optimization that launched Inspector 6, is now part of Mailchimp.
Machine Learning and Business Rules
Content Optimizer generates scorecards that reflect the content quality and number of best practices for each category of analysis, such as immobility and design. The content scorecard is available to all users. Premium users receive actionable suggestions to improve content.
“Our north star for solving this problem is to improve campaign performance. “If we can improve campaign performance by 10% across our entire user base, this will result in 190,000,000 additional online visits for our customers,” Wolf said.
Machine learning is an important component of Content Optimizer. A pipeline of ML models is used behind the scenes to analyze and parse different parts of marketing emails and predict their outcomes.
The first set of models is used to extract features from the content. These include the style of writing, the message, the layout, and the images that tell the story.
These features are then used as input for the next set of machine-learning models that attempt to predict the quality and outcome of the campaign. Content Optimizer combines ML prediction with symbolic AI in some areas to make recommendations that are robust and more understandable.
Wolf explained that “we sometimes use a hybrid machine learning model and business rules to detect certain things.” Sometimes we found that using business rules is easier to maintain and develop than machine learning.
A “call to action” is an important component of marketing assets. The most successful call-to-action sentences begin with a verb in a specific form. The Content Optimizer team discovered that this business rule performed very well. In this case, the team used ML libraries to detect different parts of speech within CTA text. The data was then fed to a static rule-based system which evaluated its quality.
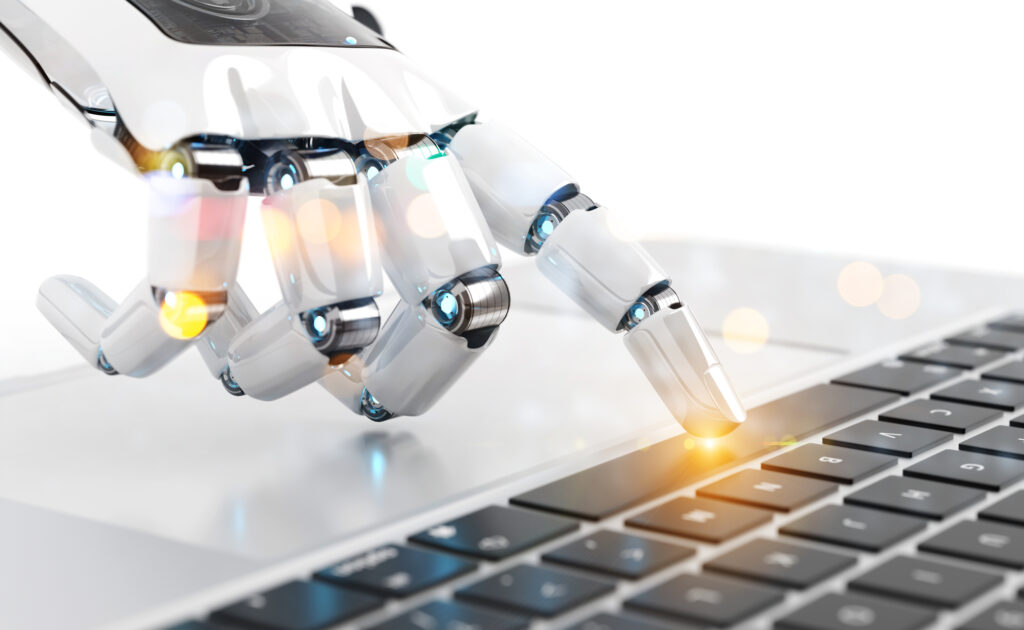
Human error
The machine learning models are valuable, but they cannot work independently yet. Mailchimp currently uses human operators to ensure that the output of Content Optimizer is in line with the recommendations made by a creative director.
Wolf explained that “we go through a predictive modeling exercise but there is a manual vetting procedure.” This is inefficient, but it is necessary for now.
Some people are against putting humans in front of AI systems. It’s sometimes called “Wizard of Oz” or pseudo-AI. In our conversation, Wolf was open and honest about this, and he believed that it would be a key factor in the success. The company does not outsource the task but instead completes it entirely with internal resources.
Wolf stated that “in the early stages of applied AI I believe the risk of losing our credibility with users by making a suggestion that is out of bounds or doesn’t make any sense is so great that we need to be extremely careful and sensitive.”
The team will fine-tune machine learning models as the Content Optimizer collects more feedback and data. They will also figure out ways to reduce their reliance on human assistance.
It takes time. It adds a labor-intensive element. Wolf added, “But it’s a field we’re willingly putting people against.”
The task may not be completely automated. A machine learning product, like any other, is a tool that can be used to solve problems faster, with greater accuracy, and for less money. Content Optimizer is a success if it helps Mailchimp improve campaign performance for its clients statistically and efficiently. AdWords is a good example of this. It’s Google’s platform for online advertising and the company’s biggest source of revenue. AdWords combines AI with human evaluation to ensure ads are relevant and in compliance with company policies.
Learn from other users
Learning from users is a key part of the product-management process. Your hypotheses are tested after a product is launched. You will usually discover pain points you underestimated or ignored, and interesting use cases that you hadn’t thought of.
The Content Optimizer, for example, showed that Mailchimp users were better at typography in general than the product team initially thought. The researchers also discovered that many marketers have difficulty writing in simple, concise language.
Wolf explained that “it’s like the collective system acts as the creative director of 14 million users, and you have to be their creative director.” Sometimes they will surprise you by what they are good at, and what they still struggle with,” Wolf said.
According to Wolf, one of the positive outcomes of the Content Optimizer is that marketers are already comfortable using the product.
“When you launch a new product, you can expect to get a lot of questions like ‘What’s this?’ or ‘How do you do it? “He said. He says that when people use Content Optimizer their conversations tend to be more about marketing than the product. This has been a pleasant surprise.
This is the goal. Wolf stated that they have taken the product, and understood it.
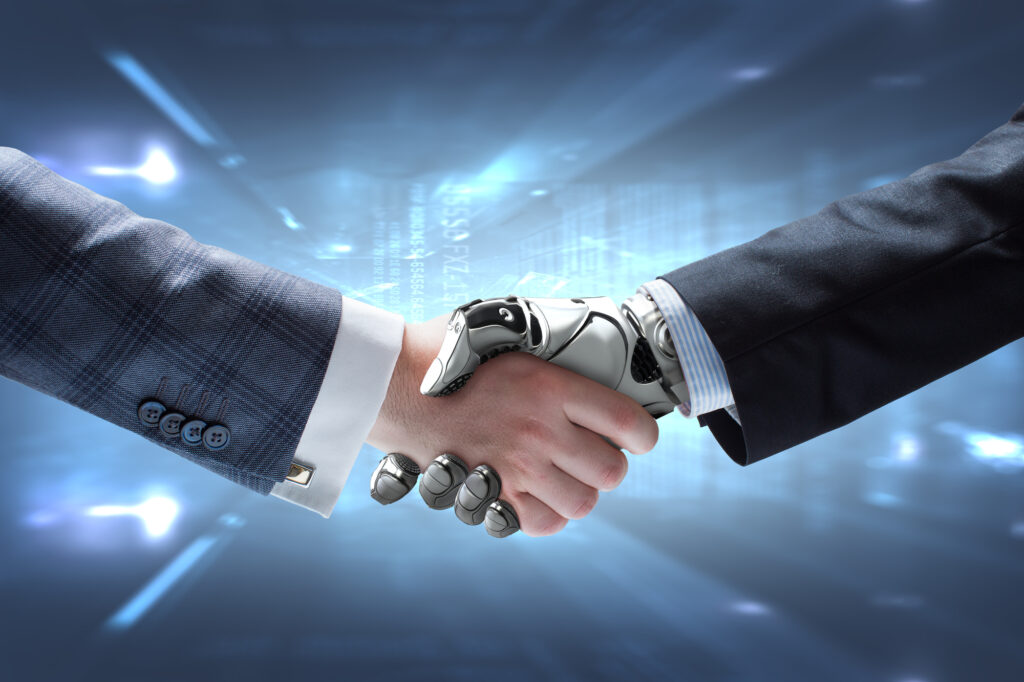
AI-powered content marketing is the future
Wolf says that his team will continue to expand Content Optimizer so it can provide more recommendations for tone, messaging, and brand consistency. It will also be expanded from email marketing into other channels, such as social media and web pages. In the future, there will be more touchpoints. The Content Optimizer tool is currently a reporting tool. However, the team intends to make it available in real-time recommendations that operate while the user edits their content.
Wolf also wants to get into computer-generated material in the future.
He says that even the most sophisticated marketers would like to spend less time creating content. “Everyone knows about cutting-edge models of copywriting generation like GPT-3. These are fantastic. How do you ensure they are on brand, on message, and optimized the same way we optimize human-generated content?”
When used alone, generative models have difficulty maintaining consistency and coherence. Wolf, however, believes that combining the Content Optimizer Pipeline with generative models such as GPT-3 will create enormous value for marketers.
“Our clients spend 28 million hours per year just writing copy, and that’s without designing or sourcing images. With some technologies, we think that this can be reduced by 80%. “We can save 22 million hours for our customers,” said he. This is staggering to me and one of the reasons I sold my business to Mailchimp was to be able to create value on such a large scale. We are excited about the future and we’re just getting started.”