Research Report
Briefly,
- Today, many companies are creating AI solutions from scratch, when they could take advantage of pre-built models, algorithms, and APIs.
- The market is filled with a growing number of options, which have been developed, refined, and tested by leading vendors.
- Many AI partners have the resources and expertise to speed up projects, reducing the risks involved in building alone.
- Learn when it makes sense to build your AI solution and how you can maximize the value of AI for your business.
The AI strategy of an organization is a key component in its overall competitive strategy. Many believe that AI will transform the competitive landscape in their industries and create new products, business models, and markets. To avoid being left behind, organizations are rushing to build their own AI solutions. Without clear business goals and strategic AI objectives, AI solutions will likely be siloed. In addition, teams end up creating internal AI capabilities and systems from scratch even when there are more efficient options available. For example, buying ready-made solutions or working with specialists.
This AI sprawl can lead to confusion within businesses, the duplication of resources, and a lack of alignment with core organizational goals. This can lead to a multitude of small AI solutions with limited impact on business. Our research indicates that less than 50% of companies are utilizing AI strategically across the entire organization. AI implemented in silos or as separate projects won’t deliver the value companies are looking for.
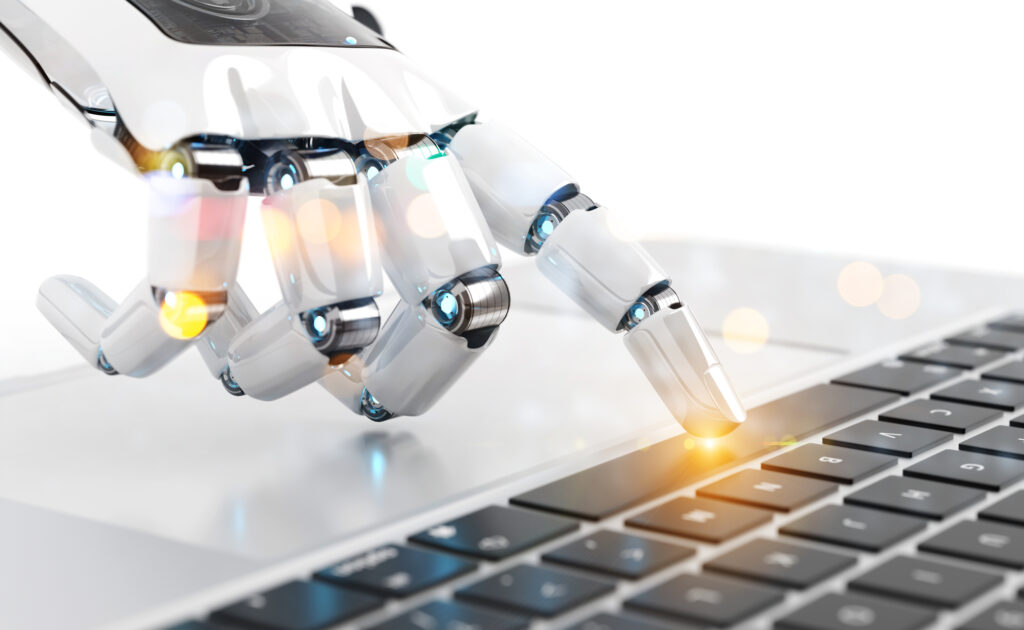
AI Strategy Options
To curb AI sprawl, and to make better decisions about implementations, organizations need to give more thought to the benefits they hope to achieve by implementing AI solutions. Three broad options are available (and hybrids of them), each suited for different scenarios.
- BUILD
- BUY
- PARTNER
Building is the process of creating an AI solution based on internal capabilities. A bank, for example, might develop a tool to predict currency demand. For this, the bank would need to hire AI and data experts to work with currency experts in-house to develop a model that could be continually improved over time.
Many teams choose the build option first, thinking that they will have the most control over what could be a differentiator in the future. Most often, though, buying or partnering makes more sense. APIs are used by small businesses to gain access to data on credit card transactions from Visa(TM), for example. This data can be used as a basis for AI solutions. Companies need to partner quickly if they don’t have the data or expertise internally. The inputs are not critical for the solution but can help speed up its execution.
As outlined below and in the tree, there are five major factors to consider when choosing an AI strategy. It’s common for companies to overlook the basic questions. This decision tree covers all bases and can help teams decide whether they want to build, partner, or buy.
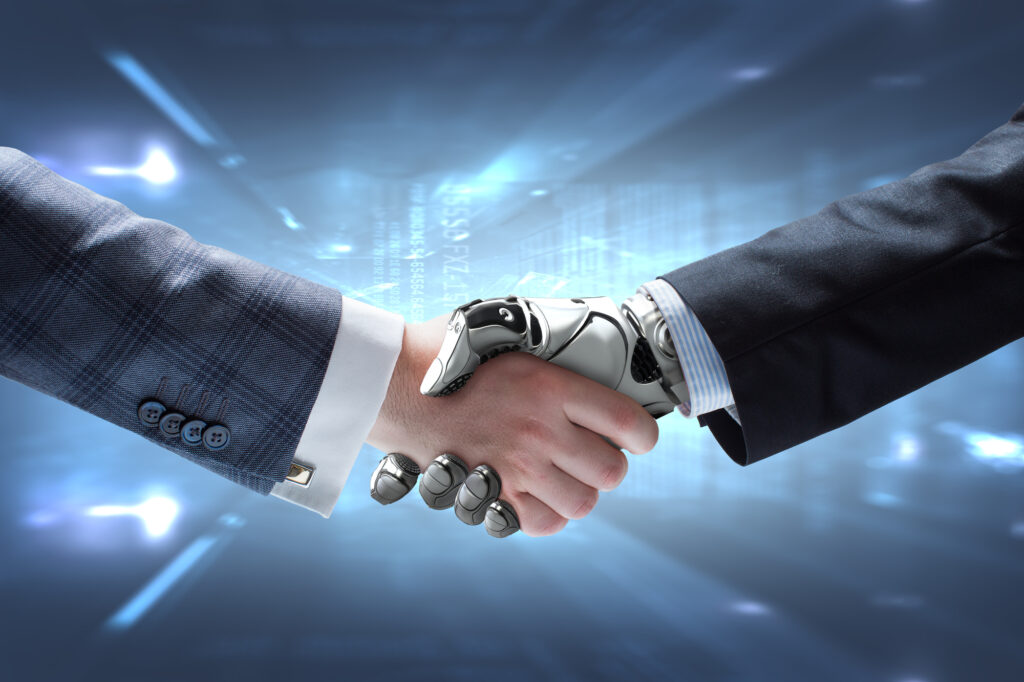
Question 1: Feasibility
Can a case study or agile pilot research be used to prove feasibility?
Companies that recognize the value of AI do just that. A quick and efficient test pilot will confirm whether the AI project is viable and if it can be scaled. In a survey of businesses that are testing new technologies, we found that a quarter were currently piloting AI-based solutions. Another quarter was evaluating or planning to pilot. If companies are not able to pilot AI solutions, research on case studies and professional consultation can also be used to demonstrate feasibility.
An innovation laboratory, or AI center for excellence, should be able to find and deliver cases of use that prove project viability in 3-6 months. These use cases need to be collected by different departments, evaluated quickly, and prioritized, or, if needed, rejected. In today’s culture of fail-fast innovation, the ability to quickly discard a business case if it is not aligned with business objectives is a mark of a successful company.
Failing to understand the business case upfront will likely result in a project taking much longer and costing more than expected, and eventually leading to disillusionment. The inability to make decisions quickly can also clog the AI pipeline, smothering potentially good initiatives and causing others to be pursued on the margins with the potential for duplication and lack of reuse.
Finally, feasibility research can also be used to determine whether there are any off-the-shelf solutions or services available that provide the desired functionality for a much lower cost than developing the capabilities in-house.
Question 2: Organizational Fit
Does the project have a real need and is it being funded?
Any AI project must be driven by demand. It is important to know the value that will be delivered, and the nature of any benefits, such as marginal or transformative. Project objectives must be aligned with the goals of the company, and stakeholders should be supportive and committed. Commitment can be seen in funding decisions but also resource allocation, communication, and flexibility within the company. Successful companies, regardless of the project they are working on, are those where senior management is fully informed and project objectives are communicated to delivery teams.
Question 3: Data
Are the data needed for the project’s intended purpose available? Are the data you require unique?
A project that uses unique data generated as part of a business model, such as customer interaction data, can become extremely valuable and strategically important. It is particularly true if data or algorithms are monetized, i.e. made available to third parties for a fee. It makes sense in such cases to build the AI service or product internally so that intellectual property rights can be retained.
If the data needed for the project are widely available on the market, such as data from Twitter ™ and other social media, then the company may want to reconsider its AI strategy and look at buying AI solutions from outside. Many services offer top-notch analysis and collect data from social media, mobile devices, or other sources. There are many options, and the prices are low. Companies can choose the “good enough solution” instead of trying to collect their data. This will slow down the AI implementation. According to our research, the lack of quality and quantity of internal data is one of the main reasons companies are unable to deploy AI.
Question 4: Strategic impact
Is your project strategic?
A project is of strategic importance if it will provide a competitive edge or transformative impact. The impact of a project will be more tactical if the goals are smaller, such as keeping up with competitors or meeting customer expectations. The project’s relevance to the unit or department implementing it and whether it is useful to other areas of the company is another factor. Even if a solution or tool can be easily adapted by other divisions, functions, or units, it may still be considered strategic.
A project of strategic importance may be a good candidate for internal development if the company has sufficient capabilities. (See Question #5) The lower risks and costs of pursuing a partner or buy approach are the best way to go for a tactically important project.
Three AI operating models
The impact of AI solutions on a company’s strategic and tactical goals may differ depending on the model of operation that each company uses to implement AI projects. Most companies tend to use one of three operating models:
- Distributed: Highly distributed working patterns are a sign of mature technologies, tools, and usage styles. Data scientists can work in loosely-connected groups and be productive with the resources they have at their disposal. They also know how to reuse and provide knowledge and expertise for the larger business.
- Centralized: On the other end of the spectrum are highly centralized models, where all data science and AI are carried out by a team specialized in this area. Local groups who want to make use of the technology consult with the center on all development aspects. This model is efficient but can be a bottleneck for growth and value in large companies.
- Hub and Spoke is a good model to use when you want to prepare yourself for the journey of maturity. It helps to drive standards, tools, and methods and guides the prioritization as the organization grows and matures to support technology. The hub is made up of specialists from the business units and people who are part of the hub.
Question #5: What are your capabilities?
Do you have the necessary technical capabilities on-site?
If AI is considered strategic, the final question is whether or not the organization can deliver it. If yes, then developing internally is usually a good idea. If speed is important and mobilizing internal resources would take too much time, it may be better to use a vendor’s solution or partner. In these situations, acquiring a startup with the right capabilities and solution could be an option.
The question becomes, if the required capabilities aren’t available, whether to build them in-house, purchase a ready-made solution, or hire a partner with specialist expertise to assist you. Many organizations struggle to find and hire the best AI talent.
It is possible to adopt a hybrid approach, where the company can rely on partners or buy components off the shelf (to achieve better results faster and with less risk), while simultaneously building a capability capable of taking on more heavy lifting in future projects.
Companies should, in all cases, make sure they’re not reinventing anything that is already commoditized, and that can be purchased at a reasonable price on the market.
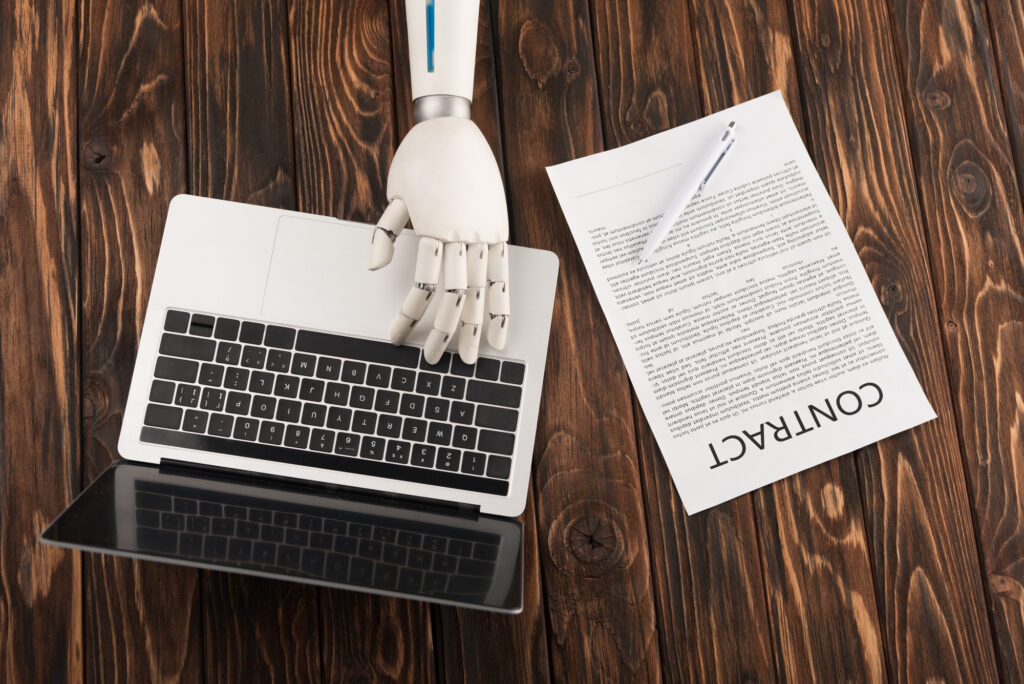
Make the right decision
What AI strategy should be chosen? Few organizations can create value by building AI capabilities internally. Often, leveraging existing solutions and abilities created by others can achieve the desired result more quickly and cost-effectively. For the same results from the building, companies will need to invest more money, time, and effort. This only makes sense when the project is strategic for them.
The best way to invest is in two different ways.
- Integrate what is already available to get on the market faster. Profit from the innovation wave and others’ investments.
- Build the capabilities that will help you to differentiate and create value in the future.
The common sense approach to AI will help organizations maximize their AI value and save time, effort, and money.