You may have noticed that people in your social network are using AI to create and share original artwork. You might have seen selfies with aesthetically altered backgrounds that mimic Renaissance art or include surrealist scenes. This technology, which has become “viral”, is known as generative artificial intelligence.
It is almost a miracle to the end user that generative AI can produce 100% original responses based on unique human inputs – from words to visualize to scripts to write! The “Christmas miracle” is possible because the technology hides the inner workings (which rely on sophisticated analysis and heavy data crunching) and only shows the results.
We’re revealing the AI innovation that has been making headlines for the past few months: generative AI. What is the secret behind generative AI’s magic? Decode this fascinating technology.
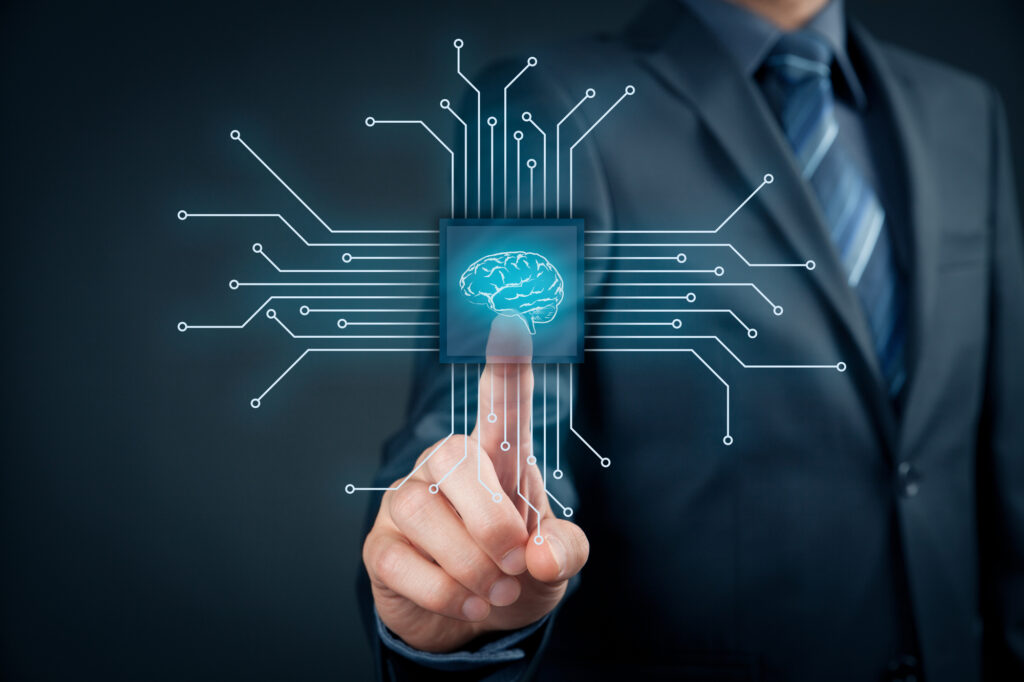
What is Generative AI (Generative Artificial Intelligence)? Definition and Meaning
Generative AI is a type of AI that generates new materials, like literature, graphics, and music. These systems use machine learning to produce new material that is similar to the examples used for training.
This is a general term for unattended or semi-attended methods of machine learning that enable computers to use existing data such as words, audio and video files, images, and even code, to create new content. It is the goal to create artifacts that are unique and appear authentic.
Gartner predicts that generative AI will change digital product development, among others. It will improve the quality, performance, and accessibility of digital products while decreasing their time to market. It is one of the many commercial advantages that generative AI offers, aside from its magical qualities. Technology is especially important in creative disciplines such as marketing and design.
How does Generative AI work?
Generative AI refers to any type of artificial Intelligence that creates new digital imagery, video or audio, text or code using unsupervised learning. The inner workings of generative AI can differ from one solution or another. There are a few things that remain the same, regardless of how gen-AI is packaged.
It is different from discriminatory AI which classifies inputs. This is what “discriminatory AI” means in this case. A discriminating learning algorithm’s goal would be to judge incoming inputs using what it has learned through training. A generative AI model, on the other hand, is designed to generate synthetic data.
In the training phase of these AI models, only a limited number of parameters are provided. This strategy essentially challenges the model to form its judgments about the most important characteristics of the data.
Three types of AI-based technology are available:
- Generative adversarial networks or GANs: Technologies that can generate visual or multi-media outputs using both language and image inputs.
- Transformer-based Models: Generative Pre-Trained language models (GPTs) can leverage Internet-driven data to produce textual materials, including website articles, news releases, and whitepapers.
- Variational Auto-Encoders: The encoder compresses the inputs, and the decoder reproduces the information.
Semi-supervised AI is a common feature of Generative AI, and GANs in particular. Semi-supervised AI learning uses both labeled examples for supervised and unlabeled material for unsupervised training. Unlabeled data can be used to develop systems that can create models of prediction beyond what is possible with labeled data.
It is important to automate repetitive tasks that are part and parcel of creative exercises.
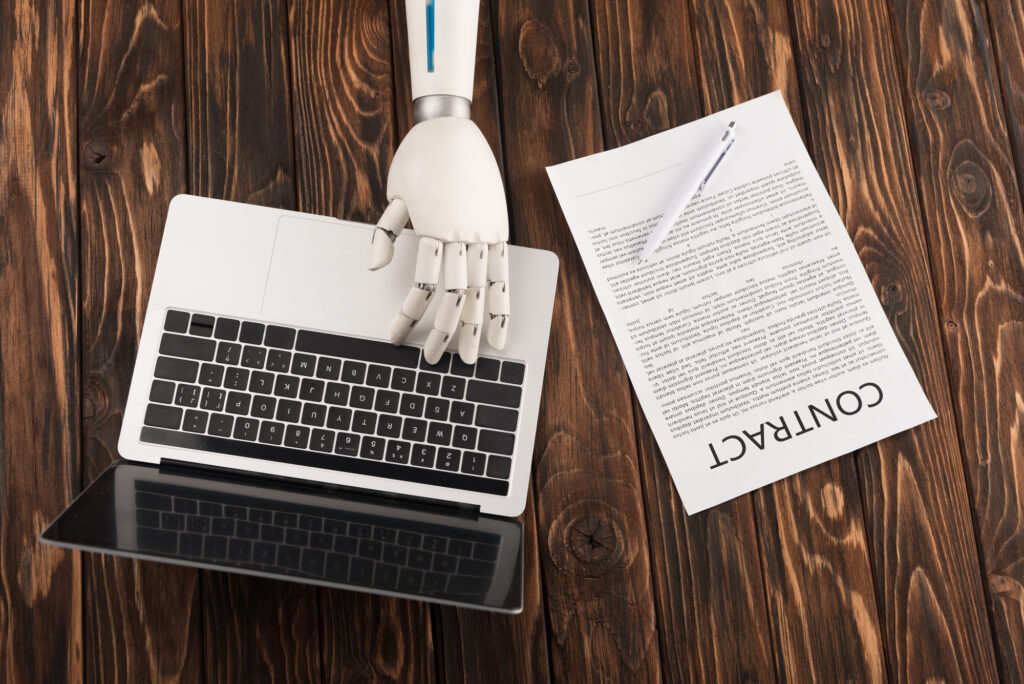
What are the top uses of Generative AI? Top Use Cases
The most promising applications of generative AI are:
1. Image Generation for Illustrations
Individuals can convert text into realistic graphics using generative AI. This is done based on the context, subject, or location. These graphic elements are important for strategic purposes, such as creating marketing campaigns.
2. Image-to-photo conversion
It is possible to create a realistic representation from a simple sketch or picture. It can be used in many ways, including map design and visualizing X-ray results. This particular use case of generative AI is very important for the health sector.
3. Image-to-image generation
This includes changing an image’s outer characteristics such as color, shape, material, or texture while maintaining its core properties. This could be done by converting a daytime photograph to a night-time one. This can be used in retail, video surveillance, and other sectors.
4. Music Experience Optimization
Audio development technologies can be used to create new audio content for advertising and other creative purposes. The AI-generated generative can produce audio clips that enhance the music listening experience on other platforms such as Spotify or social media.
5. Text generation
Generative AI is used in the gaming, marketing, and communication sectors to create dialogues, headlines, and ads. These capabilities can be used to create product details, blogs, and social media material, or in real-time chats with customers.
6. Equipment design
It can create machine parts and subassemblies. It can optimize designs for materials efficiency, clarity, and manufacturing efficiency. It is possible to feed a design into a 3D printer and get a 100% automatic part – it’s a real miracle!
7. Coding
The ability of generative AI to generate code automatically without human intervention is another use of the technology. Both professionals and non-technical people can develop code. This approach represents the next evolution in no-code app development.
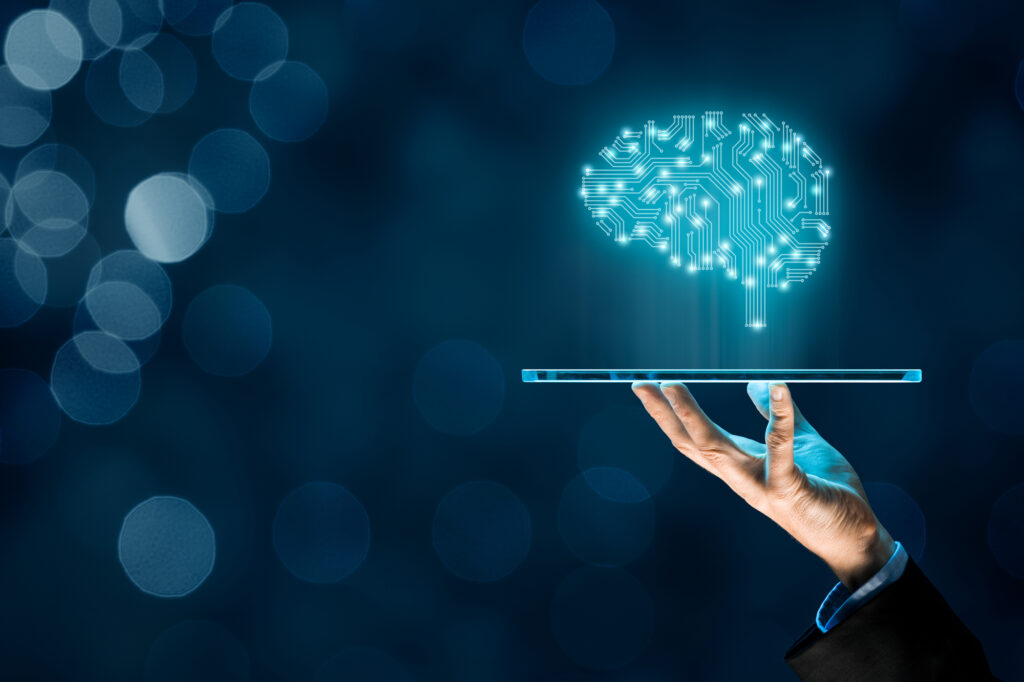
Will Generative AI replace human workers?
Some people worry about AI systems that generate fiction or create art. This leads to a broader discussion about the limits of technology and how it affects human life. Some people may view generative AI tools as task replacements, even though such technologies often include a “human-in-the-loop” component. This could lead to the creation of new jobs.
According to projections, AI is expected to boost the global economy by $15.7 trillion or 26%. Studies indicate that, even though AI may automate certain industries in the future, any job losses that result from automation will be more than offset in the long term. The larger economic impact these new technologies can create is the reason for this. Gartner says that businesses can gain a competitive advantage by using generative AI right away. They should adjust their workforce dynamics, processes, and tools.
What are the challenges of Generative Artificial Intelligence?
Generative AI may indeed seem like an amazing Christmas miracle at first, but it has its pitfalls. It is difficult to control. Since generative AI can learn on its own, it is hard to predict and regulate. The results are often far below or even opposite of expectations.
A large amount of data must be collected to train algorithms. You will get repetitive results if you only have limited data. Some applications raise questions about privacy and the ethical implications of artificial intelligence.
The hype surrounding generative AI is widespread, and it can be difficult to determine what business outcomes are realistic. Shortly, the biggest challenge is to examine the current and most popular AI frameworks to fully understand the scope and range of this emerging technology and then transform it into measurable results.